AI INFRASTRUCTURE ALLIANCE
Building the Canonical Stack for Machine Learning
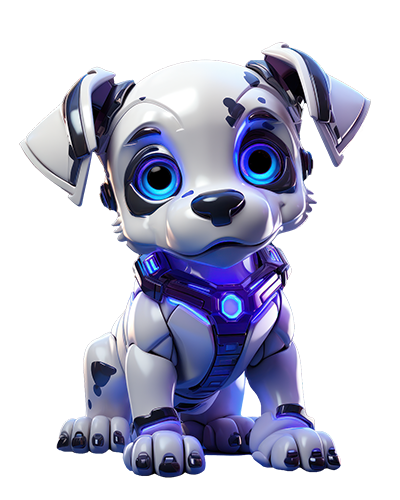
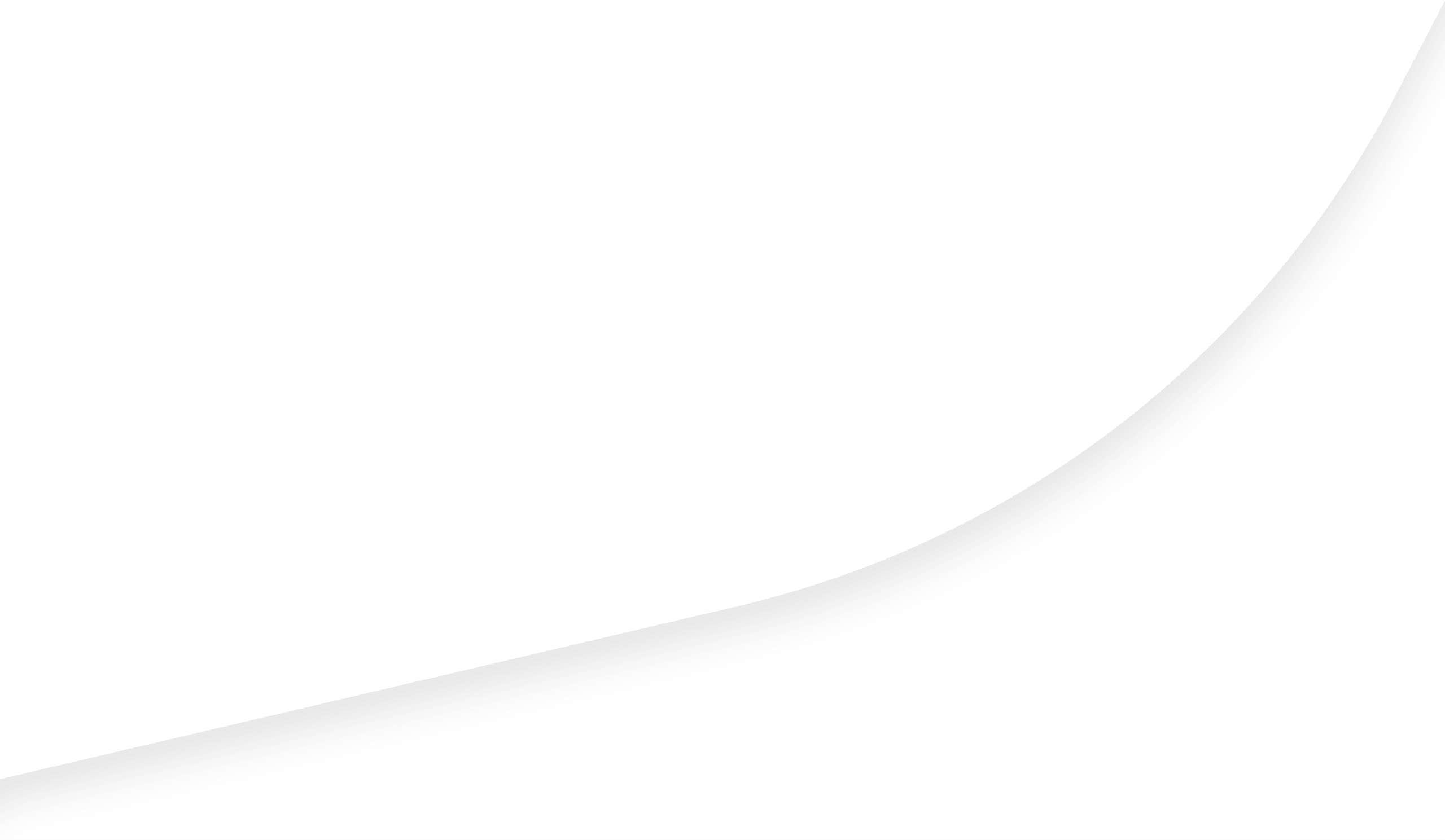
Our Work
At the AI Infrastructure Alliance, we’re dedicated to bringing together the essential building blocks for the Artificial Intelligence applications of today and tomorrow.
Right now, we’re seeing the evolution of a Canonical Stack (CS) for machine learning. It’s coming together through the efforts of many different people, projects and organizations. No one group can do it alone. That’s why we’ve created the Alliance to act as a focal point that brings together many different groups in one place.
The Alliance and its members bring striking clarity to this quickly developing field by highlighting the strongest platforms and showing how different components of a complete enterprise machine learning stack can and should interoperate. We deliver essential reports and research, virtual events packed with fantastic speakers and visual graphics that make sense of an ever-changing landscape.
Download the Enterprise Generative AI Adoption Report
Oct 2023
Our biggest report of the year covers the wide world of agents, large language models and smart apps. This massive guide dives deep into the next-gen emerging stack of AI, prompt engineering, open source and closed source generative models, common app design patterns, legal challenges, LLM logic and reasoning and more.
Get it now. FREE.
AI Landscape
Check out our constantly updated AI Landscape Graphic that shows the full range of capabilities for major MLOps tools instead of just pigeonholing them into a single box that highlights only one aspect of their primary characteristics.
Today’s MLOps tooling offers a broad sweep of possibilities for data engineering and data science teams. You can’t easily see those capabilities in typical graphics that show a bunch of logos so we’ve engineered a better info-graphic to let you quickly figure out if a tool does what you need now.
Events – Past and Future
Check here for our upcoming events and to watch videos from past events. We put on 3 to 4 major events every year and they’re packed with fantastic speakers from across the AI/ML ecosystem.
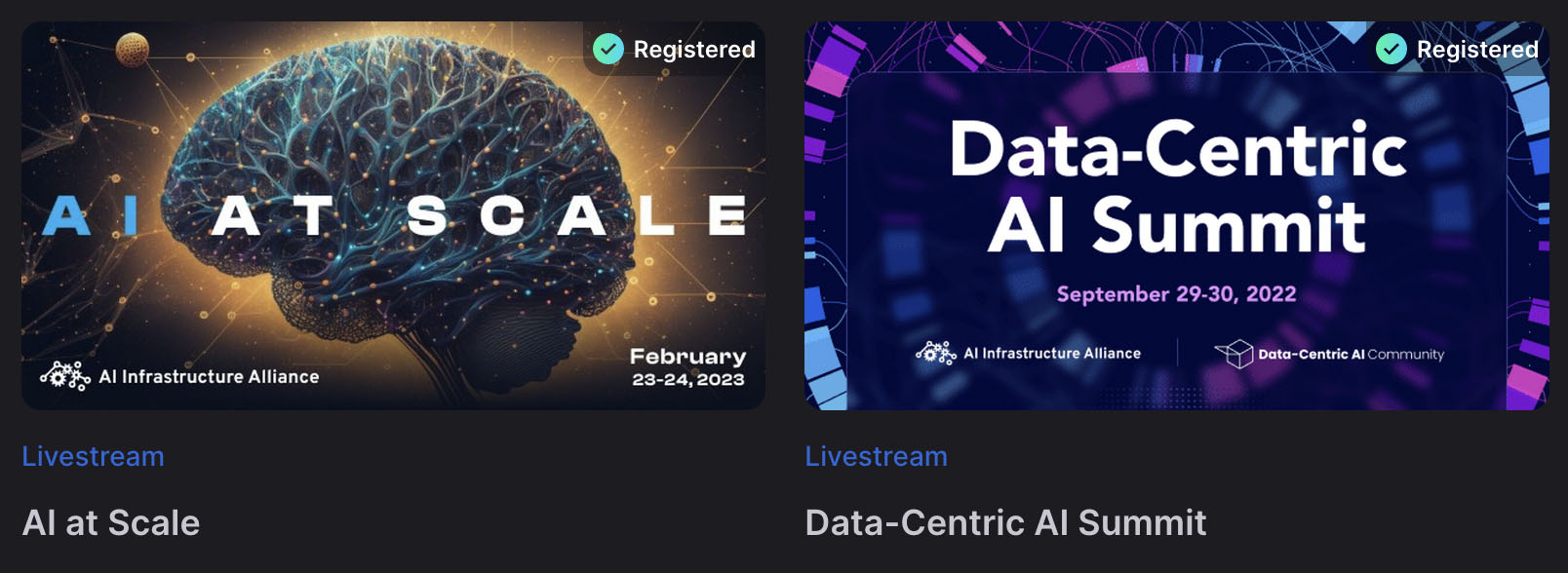
MEMBERS
ARTICLES
How To Fine-Tune Hugging Face Transformers on a Custom Dataset
In this article, we will take a look at some of the Hugging Face Transformers library features, in order to fine-tune our model on a custom dataset. The Hugging Face library provides easy-to-use APIs to download, train, and infer state-of-the-art pre-trained models...
AI Quality Management: Key Processes and Tools, Part 1
Achieving high AI Quality requires the right combination of people, processes, and tools. In the post, “What is AI Quality?” we defined what AI Quality is and how it is key to solving critical challenges facing AI today. That is, AI Quality is the set...
Shelf Engine’s CEO On Disruptive Innovation Without Disruptive Adoption and the AI-Driven Future of Grocery Retail
Stefan Kalb is on a mission to eliminate food waste and revolutionize the grocery business. Shelf Engine – the company he co-founded and leads as CEO – has notched an impressive track record since its founding in 2016, diverting over 4.5 million pounds of food waste...
The Double-Edged Sword of Generative AI: Understanding & Navigating Risks in the Enterprise Realm
Executive Summary Generative AI models and LLMs, while offering significant potential for automating tasks and boosting productivity, present risks such as confidentiality breaches, intellectual property infringement, and data privacy violations that CXOs must...
Feature Engineering for Fraud Detection
Introduction Fraud detection is critical in keeping remediating fraud and services safe and functional. First and foremost, it helps to protect businesses and individuals from financial loss. By identifying potential instances of fraud, companies can take steps to...
Machine Learning at the Edge: Elements Needed for Scale
Learn about the elements you need to build an efficient, scalable edge ML architecture. There are four components that can help bring order to the chaos that is running ML at the edge and allow you to build an efficient, scalable edge ML architecture: Central...
Connect with Us
Follow US