AI INFRASTRUCTURE ALLIANCE
Building the Canonical Stack for Machine Learning
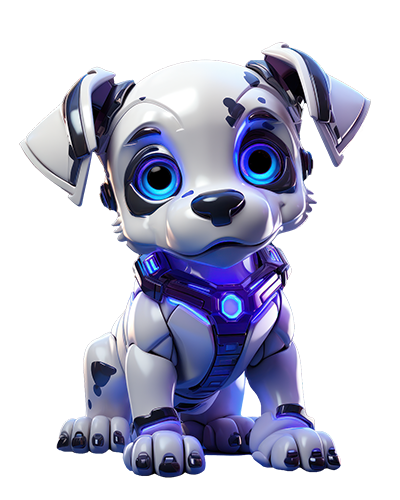
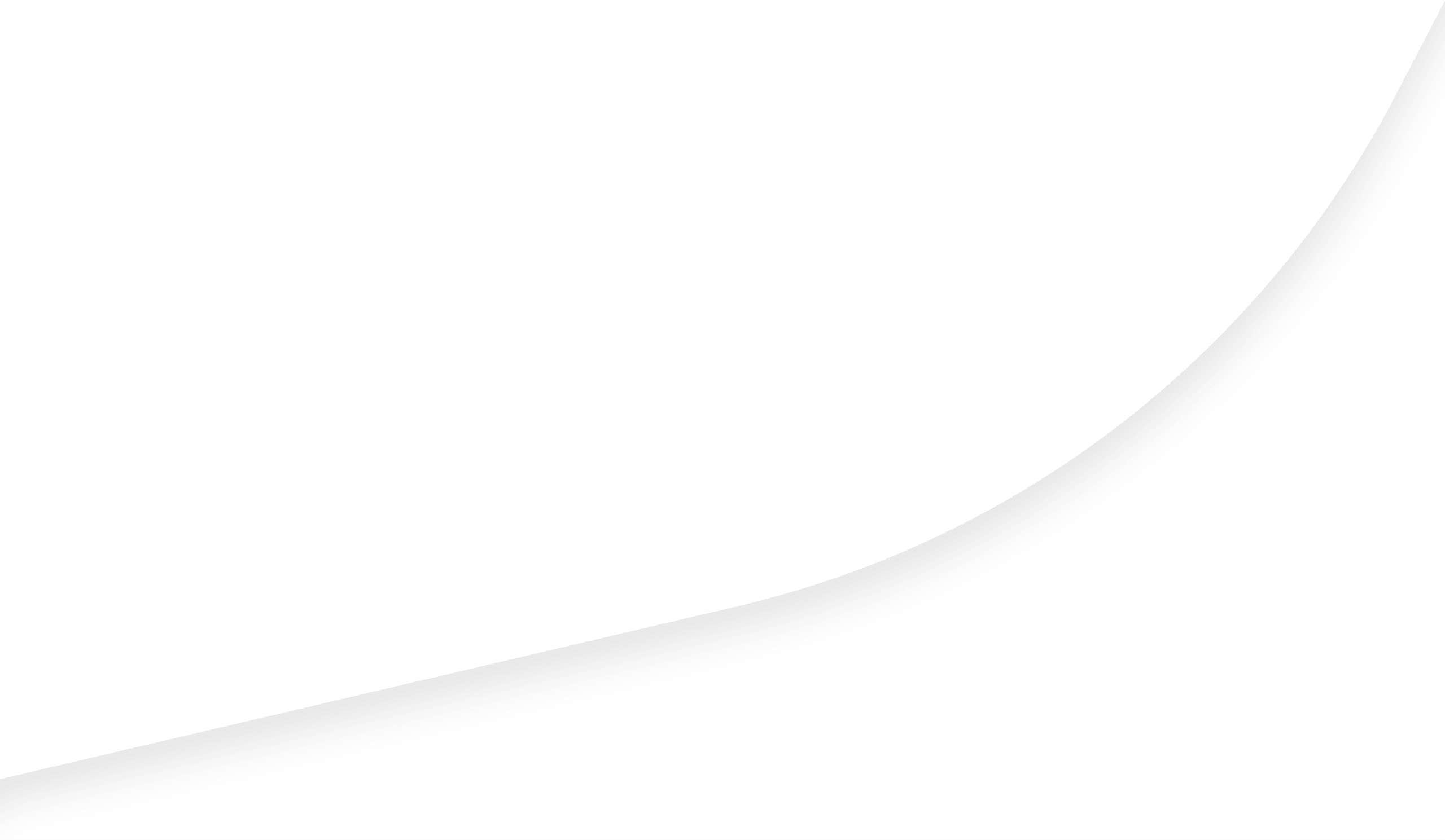
Our Work
At the AI Infrastructure Alliance, we’re dedicated to bringing together the essential building blocks for the Artificial Intelligence applications of today and tomorrow.
Right now, we’re seeing the evolution of a Canonical Stack (CS) for machine learning. It’s coming together through the efforts of many different people, projects and organizations. No one group can do it alone. That’s why we’ve created the Alliance to act as a focal point that brings together many different groups in one place.
The Alliance and its members bring striking clarity to this quickly developing field by highlighting the strongest platforms and showing how different components of a complete enterprise machine learning stack can and should interoperate. We deliver essential reports and research, virtual events packed with fantastic speakers and visual graphics that make sense of an ever-changing landscape.
Download the Enterprise Generative AI Adoption Report
Oct 2023
Our biggest report of the year covers the wide world of agents, large language models and smart apps. This massive guide dives deep into the next-gen emerging stack of AI, prompt engineering, open source and closed source generative models, common app design patterns, legal challenges, LLM logic and reasoning and more.
Get it now. FREE.
AI Landscape
Check out our constantly updated AI Landscape Graphic that shows the full range of capabilities for major MLOps tools instead of just pigeonholing them into a single box that highlights only one aspect of their primary characteristics.
Today’s MLOps tooling offers a broad sweep of possibilities for data engineering and data science teams. You can’t easily see those capabilities in typical graphics that show a bunch of logos so we’ve engineered a better info-graphic to let you quickly figure out if a tool does what you need now.
Events – Past and Future
Check here for our upcoming events and to watch videos from past events. We put on 3 to 4 major events every year and they’re packed with fantastic speakers from across the AI/ML ecosystem.
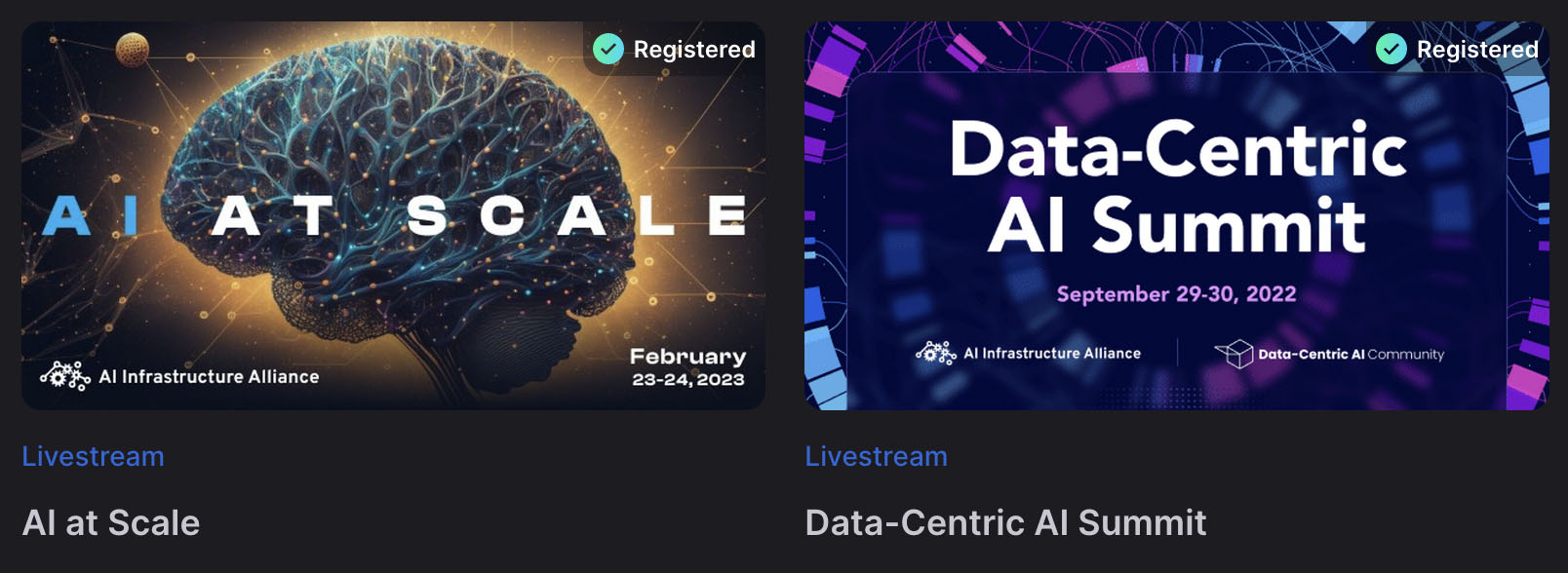
MEMBERS
ARTICLES
Are DevOps and MLOps the Same Thing?
An overview of what DevOps and MLOps have in common and what are their differences (if any). About ten years ago, the community realized that there was a barrier between the delivery and operations teams. On the one hand, there was the development team, which was...
Data Drift Detection
Data drift occurs when a model sees production data that differs from its training data. If a model is asked to make a prediction based upon drifted data, the model is unlikely to achieve its reported performance. This phenomenon happens because during training, a...
5 Reasons your ML Model isn’t Performing Well in Production
We’ve all been there. You’ve spent months working on your ML model: testing various feature combinations, different model architectures, and fine-tuning the hyperparameters until finally, your model is ready! Maybe a few more optimizations to further improve the...
How to Build a Better AI For Your Business – with Hyun Kim
Is it possible to make artificial intelligence more accessible to companies, both large and small? Our latest episode is with Hyun Kim, Co-Founder and CEO of Superb AI, that is aiming to solve this challenge and building a platform that aims to make shipping AI models...
Autoscaling Pachyderm Pipelines on AWS with Cluster & Fargate
Some steps in your machine learning pipelines may need a lot of extra horsepower to finish in a reasonable amount of time. In Pachyderm, the number of pipeline workers can be increased manually using the parallelism_spec, but that still requires the underlying compute...
Improving Your ML Datasets with Galileo, Part 1
At Galileo, we strongly believe that the key to unlocking robust models is clean, well formed datasets. Although data quality issues are prevalent in production datasets, most modern solutions aren’t built to address these problems effectively. Rather, modern...
Connect with Us
Follow US