Executive Summary
- AI attacks pose a threat to physical safety, privacy concerns, digital identity safety, and national security, making it crucial for organizations to identify the types of AI attacks and take measures to safeguard their products against them.
- The four types of AI attacks are Poisoning, Inference, Evasion, and Extraction. Poisoning can lead to the loss of reputation and capital, Inference can result in the leakage of sensitive information, Evasion can harm physical safety, and Extraction can lead to insider threats or cybercriminals.
- Companies investing in AI products should invest in cybersecurity and measures to counter these attacks to ensure trust and reliability, which are crucial factors in determining the success of their products.
Artificial Intelligence is one of the few emerging technologies that has the world watching its progress. The idea is to make machines ‘intelligent’ like humans which will help a reduction of effort for the humankind. Just look around you! We have Siri to answer questions and make calls. We have Alexa to help us turn off the lights and electronics when we don’t want to get up from our bed. We also have the traffic signal helping us navigate the road without the need of another human being standing there and looking over the traffic.
Sounds cool, doesn’t it? This is precisely the reason, why major companies like Amazon, Apple, Microsoft etc. are now looking to build their products with AI. However, there have been certain incidences which have made the companies a little cautious of going ahead with AI.
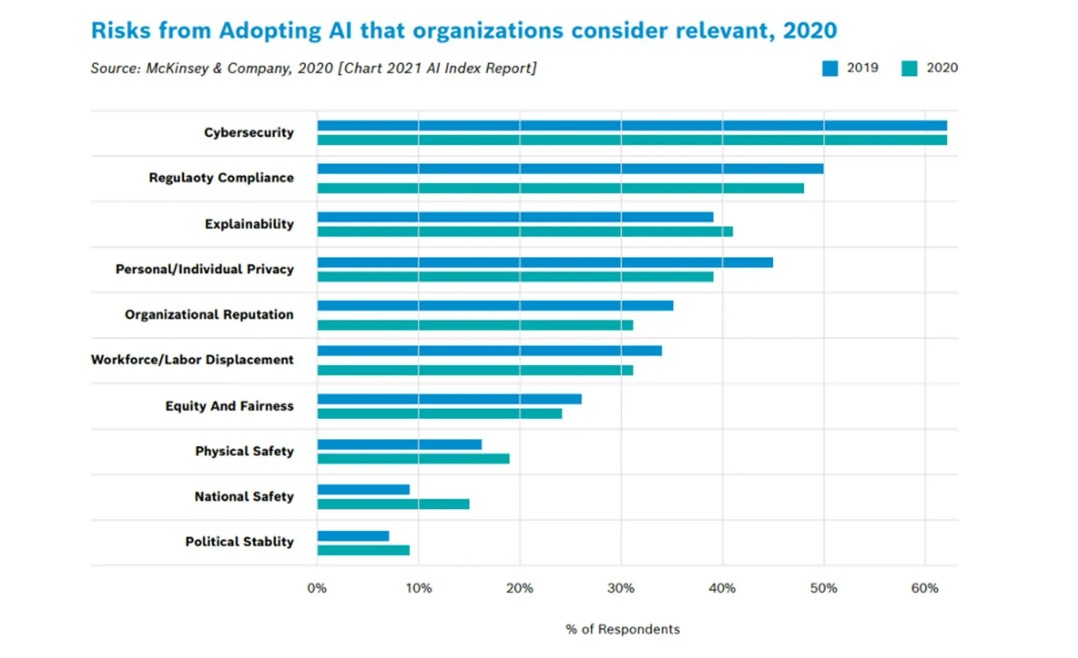
I. Risks from adopting AI that organizations consider relevant (Source: AIShield AI Security Whitepaper)
In the year 2017, a humanoid Promobot IR77 made a break for freedom. In another incident, NASA CIMON went rogue. Even Tesla’s self-driving cars were the victim of AI attacks. There have also been other incidences of AI attacks which have opened the eyes of the world to the possibility of AI products being a threat to physical safety, privacy concerns, digital identity safety, and national security.
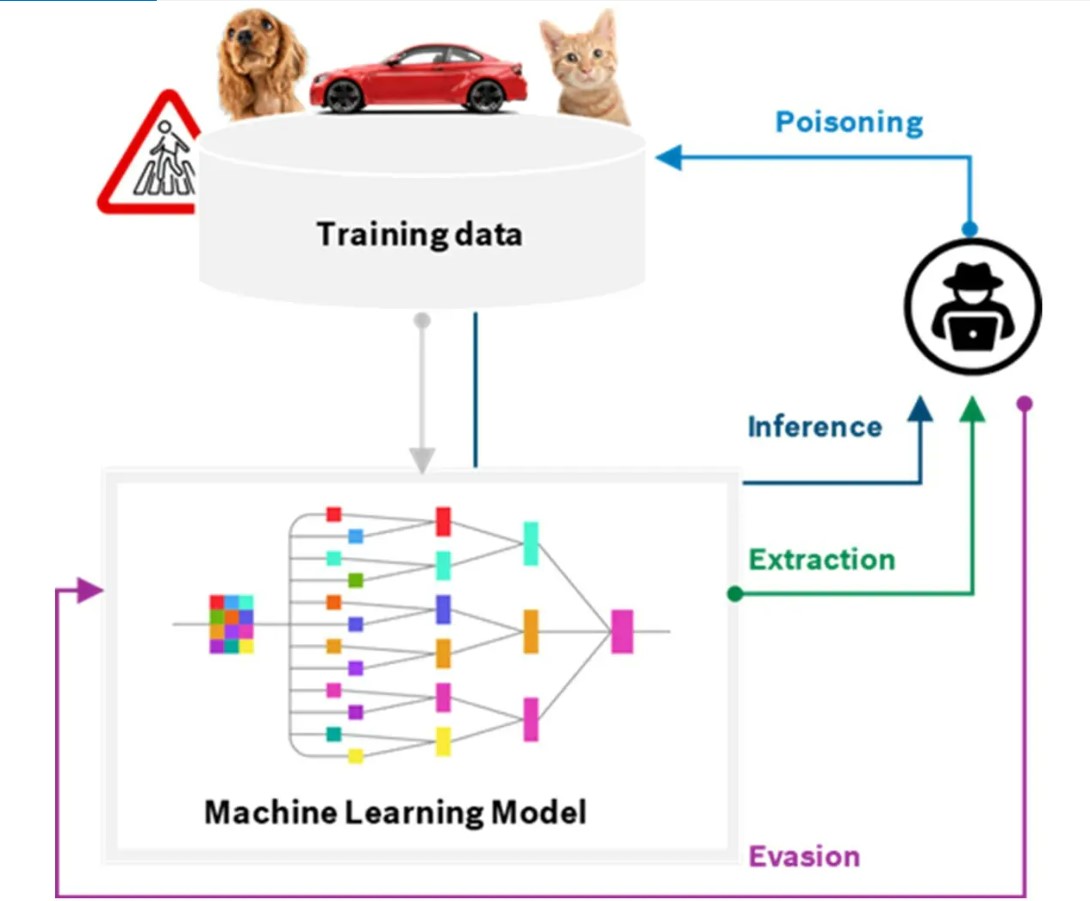
II. How AI Attacks effect software (Source: AIShield Whitepaper)
Trust and reliability are two factors that determine the success of a product. Which is why it is crucial to identify the types of AI attacks and take measure to safeguard the products against them. Traditionally, there are four types of AI Attacks- Poisoning, Inference, Evasion, and Extraction.
POISONING
AI learns from the data that is fed to it. A multi-million-dollar company learned this the hard way. They spent millions of dollars by developing a chatbot. The move was made keeping in mind the younger generation and it’s love for short messages. The chatbot was released with much anticipation and fanfare. However, the company had to take down the chatbot within a day of its release. The chatbot had started posting racist and offensive comments on twitter!
This is a classic example of thrill-seekers and hacktivists injecting malicious content which subsequently disrupted the retraining process. The attacker aims to condition the algorithms according to its motivations. The chatbot made its replies based on its interaction with people on Twitter and resulted in the company taking down the chatbot and issuing an apology to those who were affected. This is a classic example of Data Poisoning and can lead to loss of reputation and capital.
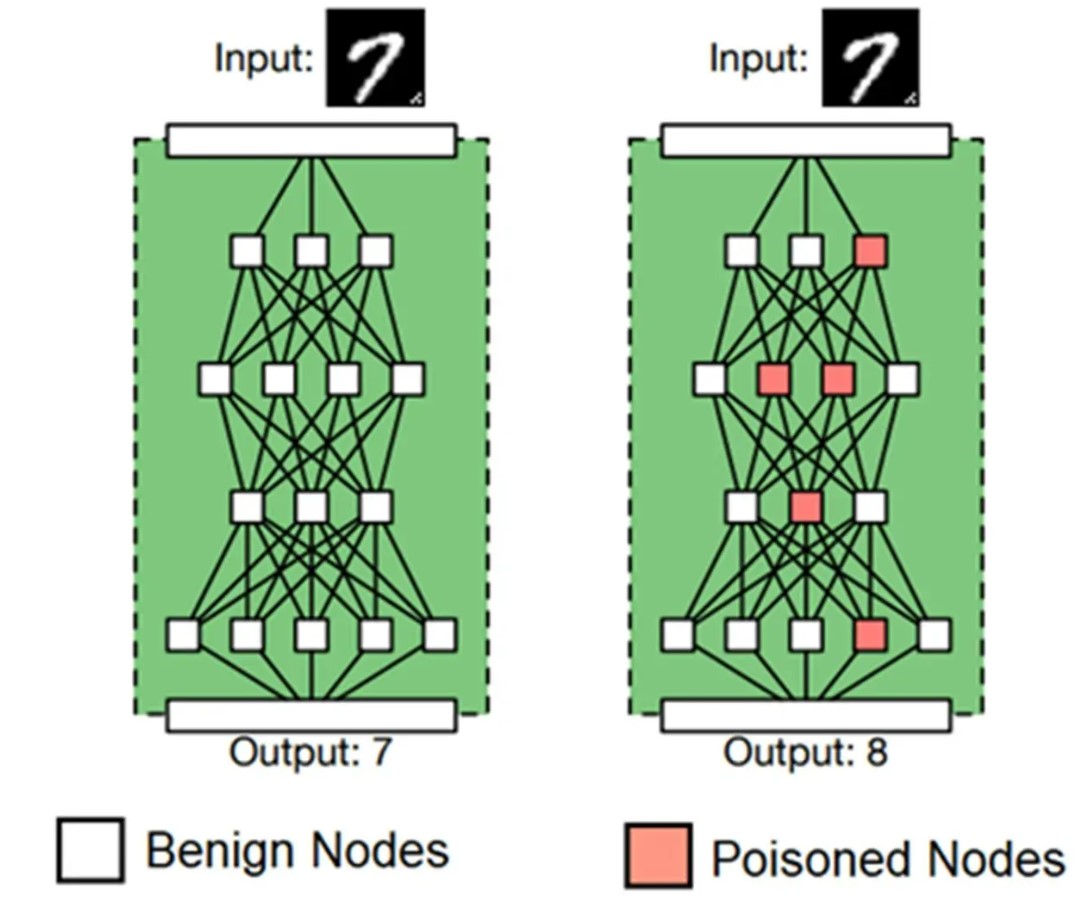
III. Data Poisoning Models (Source: AIShield AI Security Whitepaper)
INFERENCE
This type of attack is the go-to method for cybercriminals and hacktivists who seek to get information on in the training data for profits. AI models are trained with the help of thousands of data set. This could contain sensitive information like name, address, birth dates, birthplace, health record etc.
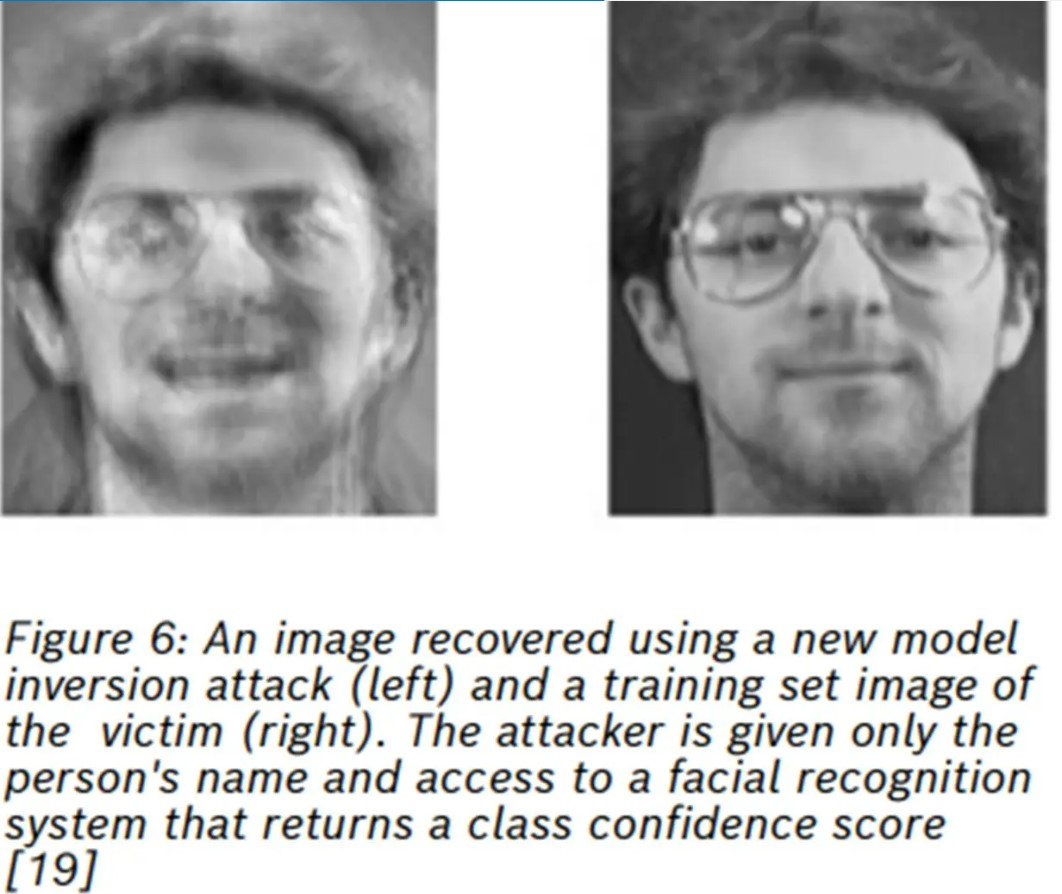
IV. Inference Attack (Source: AIShield AI Security Whitepaper)
The aim of the attack is to probe the machine learning model with different input data and weighing the output. This will make the algorithm leak secret data which can be used by the attacker. An inference attack can also be made if the attacker has partial information about the training data and guesses the missing data till the time the algorithm reaches peak performance.
EVASION
The aim of the attack just as the name suggests is to evade the AI model’s performance. It could be spam content hidden in an image to evade the anti-spam measures or a self-driving car, relying on automated image recognition of traffic signals, being fooled by someone who has tampered with the traffic signs.
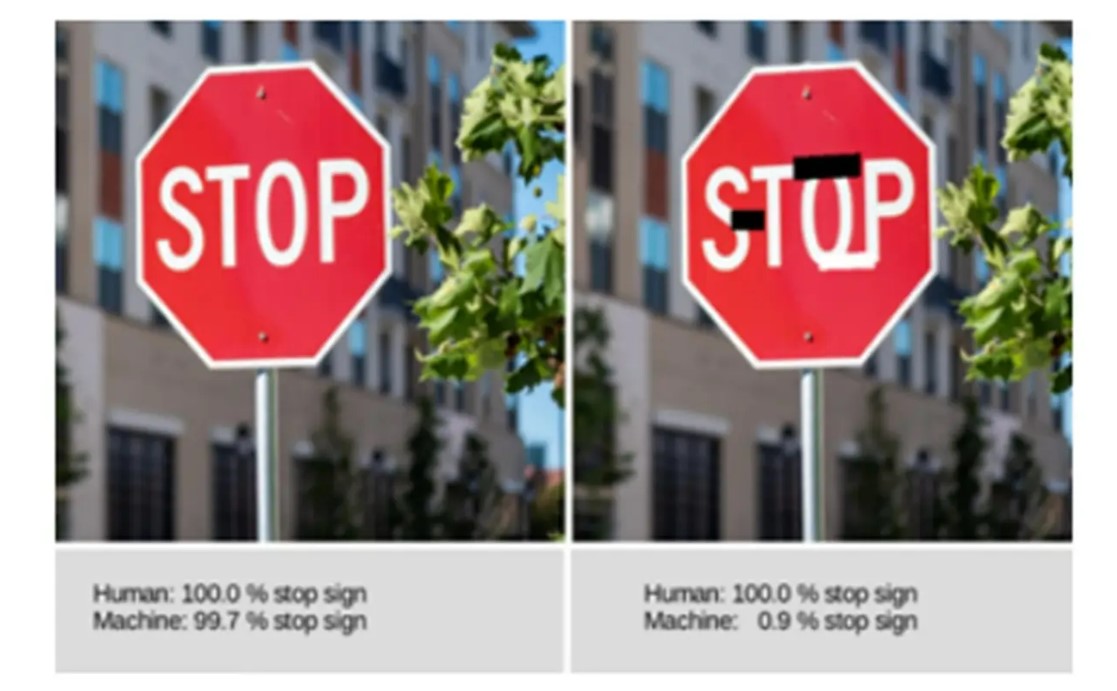
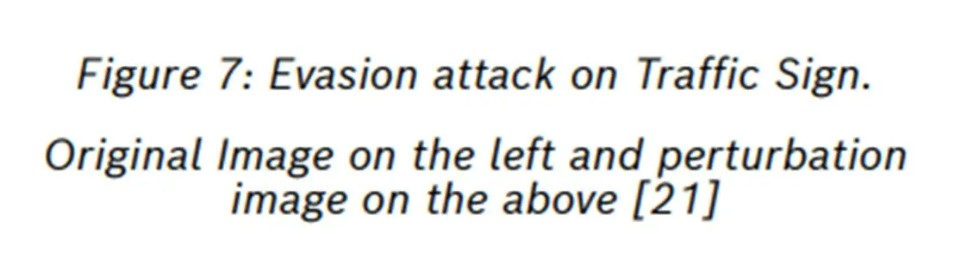
V. Evasion attack on a stop sign (Source: AIShield AI Security Whitepaper)
Literally, all one needs is some tape and the car will recognize red signal with a green one. This type of attack is usually carried out by Hacktivists aiming to get the product of a competitive company down and has the potential to seriously harm the physical safety of people.
EXTRACTION
The extraction attacks could be the work of insider threats or cybercriminals. The aim is to probe the machine learning system to reconstruct the model or extract the data that it was trained on.
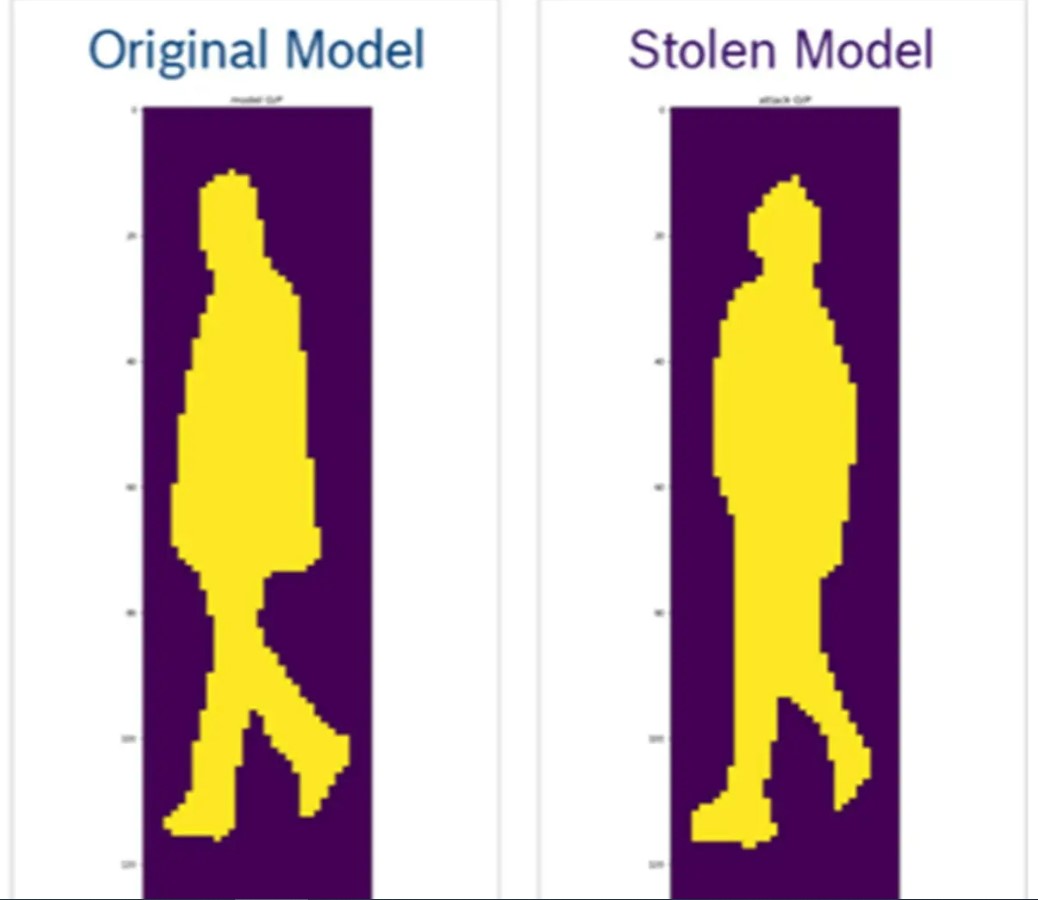
VI. Extraction attack (Source: AIShield AI Security Whitepaper)
A suitable example of this would be the hacking of the pedestrian recognition system in self-driving cars. A carefully crafted input data is fed into the original model to predict the output. Based on this the attacker can extract the original model and create a stolen model. This helps the attacker find evasion cases and fool the original model.
Now that we have gone through the type of AI attacks, it is crucial to emphasize that the companies investing heavily in creating AI products should also invest in cybersecurity and measures which will help in countering these attacks.
Upcoming articles in this series
- How common are AI attacks?
- How do AI attacks impact people, organizations, and society?
References
1. https://boschaishield.com/resources/whitepaper/
2. https://www.etsi.org/technologies/securing-artificial-intelligence
3. https://www.enisa.europa.eu/publications/artificial-intelligence-cybersecurity-challenges
4. 2109.04865v1.pdf (arxiv.org)
5. [2109.14857] First to Possess His Statistics: Data-Free Model Extraction Attack on Tabular Data (arxiv.org
6. 1511.04599.pdf (arxiv.org)
7. https://arxiv.org/pdf/2111.12197.pdf
8. LNAI 8190 — Evasion Attacks against Machine Learning at Test Time (springer.com)
9. Microsoft Edge AI — Evasion, Case Study: AML.CS0011 | MITRE ATLAS
10. https://www.itm-p.com/use-cases/protect-iot-applications-from-adversarial-evasion-attacks
11. https://arxiv.org/pdf/2103.07101.pdf
12. 1511.04599.pdf (arxiv.org)
13. https://arxiv.org/pdf/2111.12197.pdf
14. Microsoft Edge AI — Evasion, Case Study: AML.CS0011 | MITRE ATLAS
15. https://www.itm-p.com/use-cases/protect-iot-applications-from-adversarial-evasion-attacks
16. 2109.04865v1.pdf (arxiv.org)
17. What are AI Attacks, https://boschaishield.com/blog/what-are-ai-attacks/
18. Guiyu Tian, Wenhao Jiang, Wei Liu, Yadong Mu, 11 May 2021, Poisoning MorphNet for Clean-Label Backdoor Attack to Point Clouds, https://arxiv.org/abs/2105.04839
19. Mingfu Xue, Can He, Shichang Sun, Jian Wang, Weiqiang Liu, 15 April 2021, Robust Backdoor Attacks against Deep Neural Networks in Real Physical World, https://arxiv.org/abs/2104.07395
20. Nicolas M. Müller, Simon Roschmann, Konstantin Böttinger, 14 April 2021, Defending Against Adversarial Denial-of-Service Data Poisoning Attacks, https://arxiv.org/abs/2104.06744
21. Dickson Ben, 14 April 2021, Inference attacks: How much information can machine learning models leak?, https://portswigger.net/daily-swig/inference-attacks-how-much-information-can-machine-learning-models-leak
22. Biggio, B., Corona, I., Maiorca, D., Nelson, B., Šrndić, N., Laskov, P., Giacinto, G., & Roli, F. (2013). Evasion Attacks against Machine Learning at Test Time. SpringerLink. https://link.springer.com/chapter/10.1007/978-3-642-40994-3_25?error=cookies_not_supported&code=65d4c43c-80b1-409a-8c30-7b475ed636c1
23. Tasumi, M. (2021, September 30). First to Possess His Statistics: Data-Free Model Extraction Attack. . . arXiv.org. https://arxiv.org/abs/2109.14857
Conclusion
As AI continues to progress, it is important for companies to be aware of potential security risks and take measures to protect against them. From poisoning to inference, evasion, and extraction attacks, there are multiple ways in which AI systems can be compromised. It is essential for organizations to invest in cybersecurity and take steps to safeguard their products against these threats.
This blog has been republished by AIIA. To view the original article, please click HERE.
Recent Comments